Forty-seven percent of our audience reports wishing they had started networking earlier in their careers (according to our community survey in 2023). Start your journey networking with the world’s most trusted association of computing professionals.
Spread knowledge around the world
Seize opportunities to make a meaningful impact
Meet and collaborate with global leaders in computing
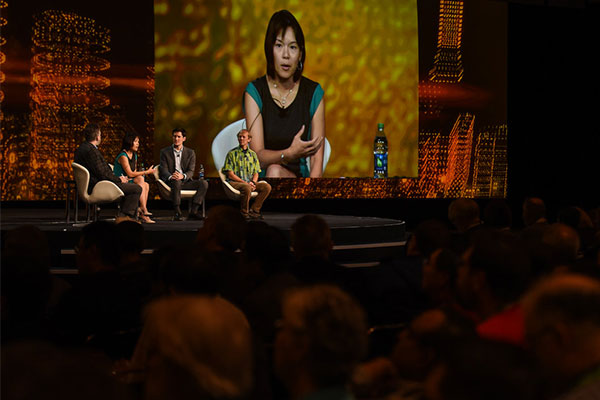
Find Connections in Your Field with Technical Communities
If you are seeking a community dedicated to your field of expertise, look to Technical Communities. Members in these communities organize conferences, engage with publications, influence the development of standards, develop programs for student activities, and create new resources with the financial backing and staff support of the IEEE Computer Society.
Technical Communities support evolving technology areas, including:
- Cloud Computing
- Computational Life Sciences
- Data Engineering
- Pattern Analysis and Machine Intelligence
- Microprocessors
- High Performance Computing (HPC)
- Software Engineering
- Explore More Focus Areas
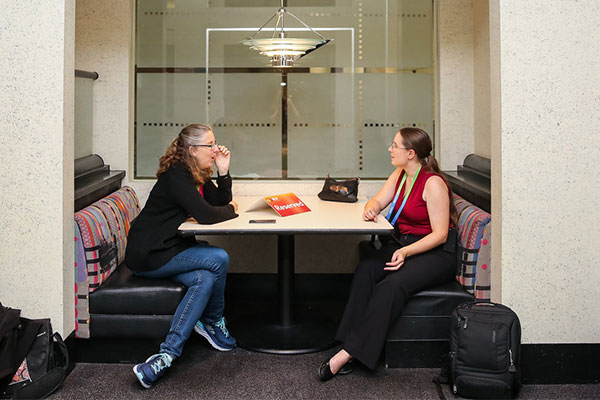
Resources for Women in Computing
IEEE Computer Society recognizes that women around the world continue to face unique challenges in pursuit of careers within computer science and engineering. We’ve compiled helpful resources that support girls and women entering computing careers and referral recommendations for groups, networking, and awards.
- Browse Resources for Women
- Celebrate Women in Computing
- Learn about Diversity & Inclusion at IEEE Computer Society
Collaborate with Sister Societies Globally
See our list of partnering organizations worldwide If you are a member of a sister society, and choose to join the IEEE Computer Society, you can deduct 10% from IEEE CS dues! Reach out to membership@computer.org with any questions. Looking to establish an agreement? Please email membership@computer.org
Local Chapters
Student and professional chapters are supported worldwide to empower the computer science and engineering community at the local level. Chapters host regular meetings for socializing, discussion of hot topics, providing guest lecturers, and even organizing community building events for mentoring early career professionals. Best of all, local chapters possess the flexibility and resources for initiating creative projects.
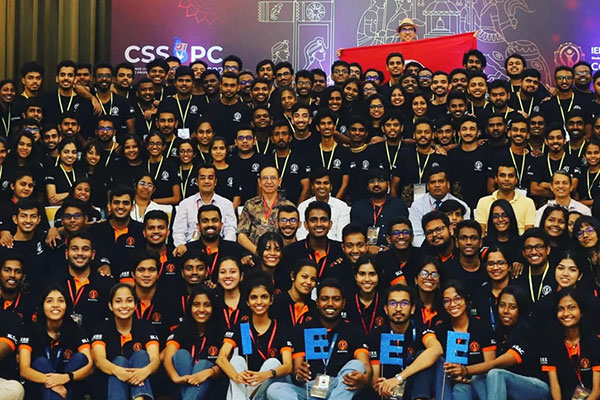